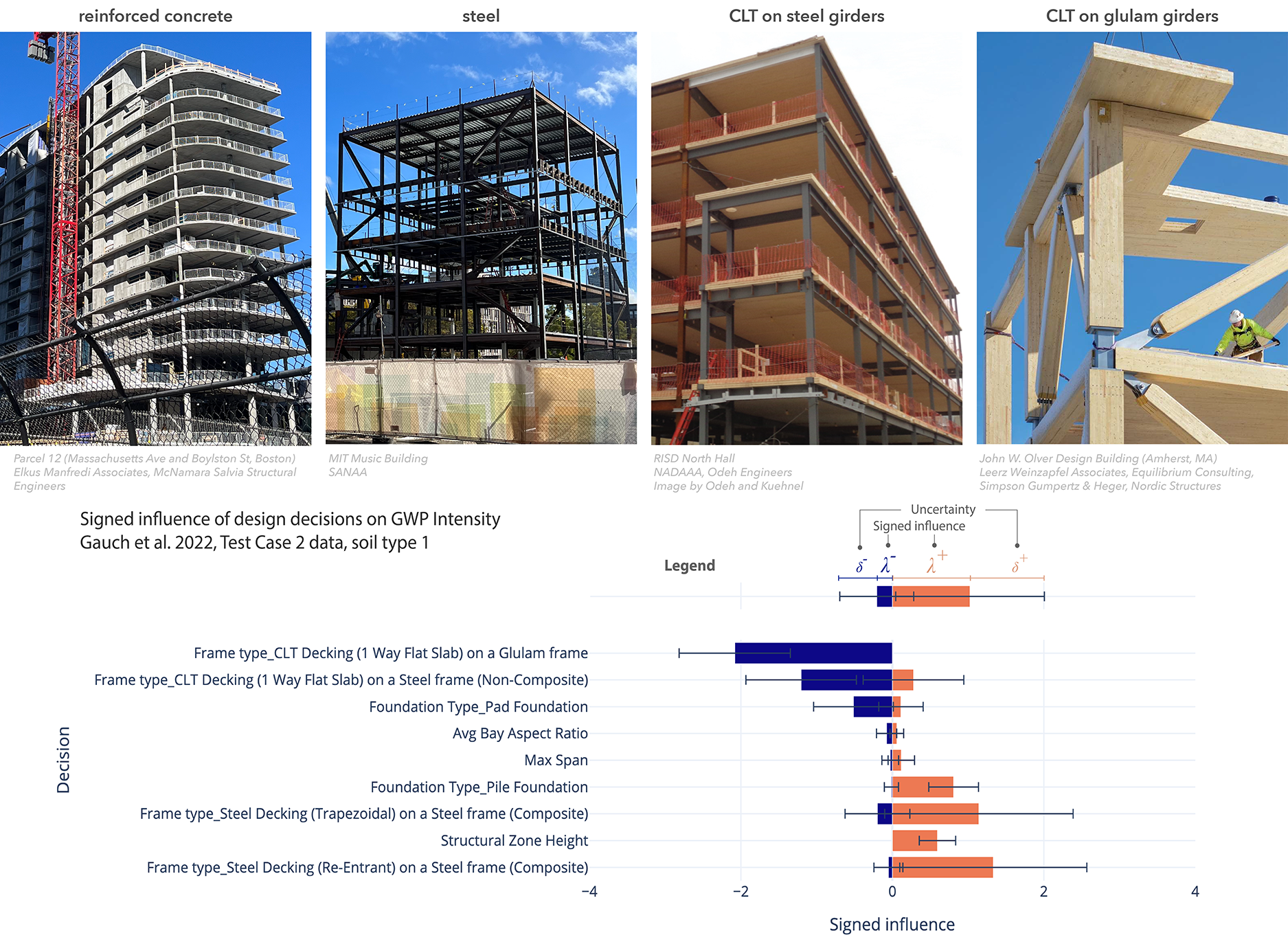
Does a timber beam or a steel truss result in a lighter structure? Between shortening the span and decreasing the load demand, which results in a more efficient structure?
Structural designers pursuing high-performance design must typically make decisions based on perceived tradeoffs. As an alternative to the extreme paradigms of deploying rules of thumb and blackbox optimization, a new paradigm of “performance-informed, human-driven design” is proposed in which designers extract data-driven insights from a provided design space to inform decision-making. The four-step computational framework entails selecting a sample representative of the design space of interest, training a machine learning model, computing gradients of the model, and computing influence metrics. Applied to the case study of a long-span structure, this paper demonstrates how this gradient-based approach can offer a data-driven way to support and augment intuitions about performance-driven design. Choice of structural typology is demonstrated to be most associated with large changes of GWP. While this insight is specific to this particular long-span design problem, its significance lies in showcasing how decision-making insights tailored to specific problems can be derived from intricate mixed-variable design domains. This outcome underscores the potency of such approaches in informing system-level design processes for low-carbon structures.
Related publications: